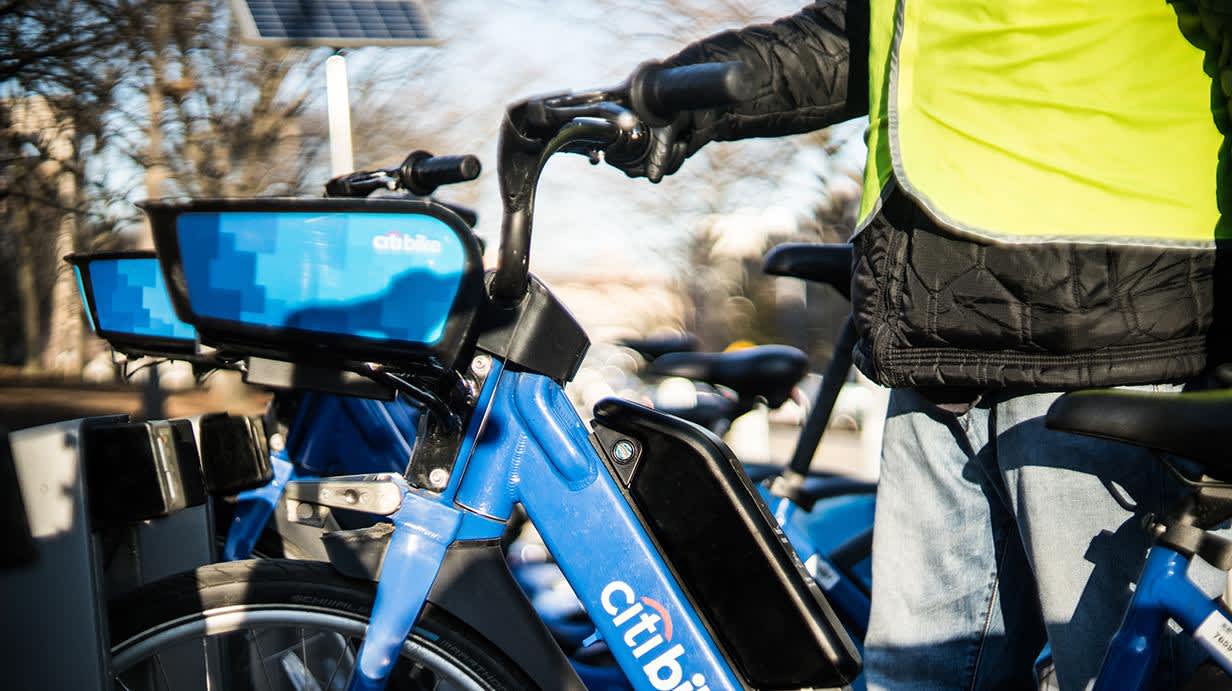
Every day in New York City, some 80,000 times on average, someone unlocks a Citi Bike from a dock. There’s no way of knowing where each of those riders is headed — over a bridge or down the block — but the sum of their individual actions rarely leads to collective harmony. Like a tidal flow, bikes pool in some neighborhoods, while others are left high and dry.
“Rebalancing” is the underappreciated, often unseen process of putting the country’s biggest bike-share program back into something approaching equilibrium. It sounds relatively simple: Take bikes from areas of low demand and move them to areas of high demand. But that seemingly basic concept requires a complex, coordinated effort involving vast amounts of real-time system data, sophisticated demand-forecast algorithms — as well as old-school, wheels-on-the-ground physical movement of bikes around the city.
Of course, Citi Bike isn’t the only transportation service facing this problem. Trucking firms are plagued by the need to return trucks without cargo, airline companies deploy high-order math to match crews with airplanes, and rental car agencies often need to move cars from one location to another. And many bike-share systems need to rebalance.
But New York’s system, one of the world’s largest and most popular, presents unique challenges. “We are truly operating at full steam every day of the week, all hours of the day, 12 months a year,” says Patrick Knoth, senior manager of strategy and operations for Lyft, which operates Citi Bike. In the city that never sleeps, the rebalancing effort doesn’t either.
A campaign of analytics and modeling
The project is guided by an energetic campaign of analytics and predictive modeling. On its surface, rebalancing bikes may seem like a fairly straightforward extension of the traveling salesman problem — an almost-century-old approach to route optimization that inspires everything from airline schedules to mail delivery routes. But the Citi Bike system is too large, nuanced, and dynamic for traditional solutions. “We’ve tried some standard approaches and found they just weren’t fast enough,” says Timon Stasko, data science manager at Lyft. “Every few minutes, our situation is changing.”
Lyft’s approach with Citi Bike doesn’t just provide a snapshot of every bike in the system — it looks forward and backward. After all, it’s not so useful to know how many bikes a station needs right now if it will take an hour to deliver bikes there. Instead, Lyft tries to anticipate what the system will look like, based on what it has looked like over the past three weeks. “We look at ridership trends in recent history,” says Stasko, “so we have a sense of how many people are probably going to leave from a station, as well as how many people are going to come to that station.” The difference between those numbers suggests how many bikes need to be moved there.
While the algorithm does include local nuances — like keeping certain neighborhoods underserved by transit well-stocked — the bulk of its decisions are oriented around one key metric: enabling the most rides. To perform that calculation, the team must forecast a few moves ahead, like a good chess player would: If a bike is moved, how many future trips will that enable? Or, by contrast, how many trips from its current station will that prevent?
Bike supply is just part of the equation. The Lyft team also has to worry about dock supply. At the end of a long commute, no rider wants to be confronted with only full docks in their neighborhood and a frantic game of urban musical chairs as they look for an empty spot to stash their ride.
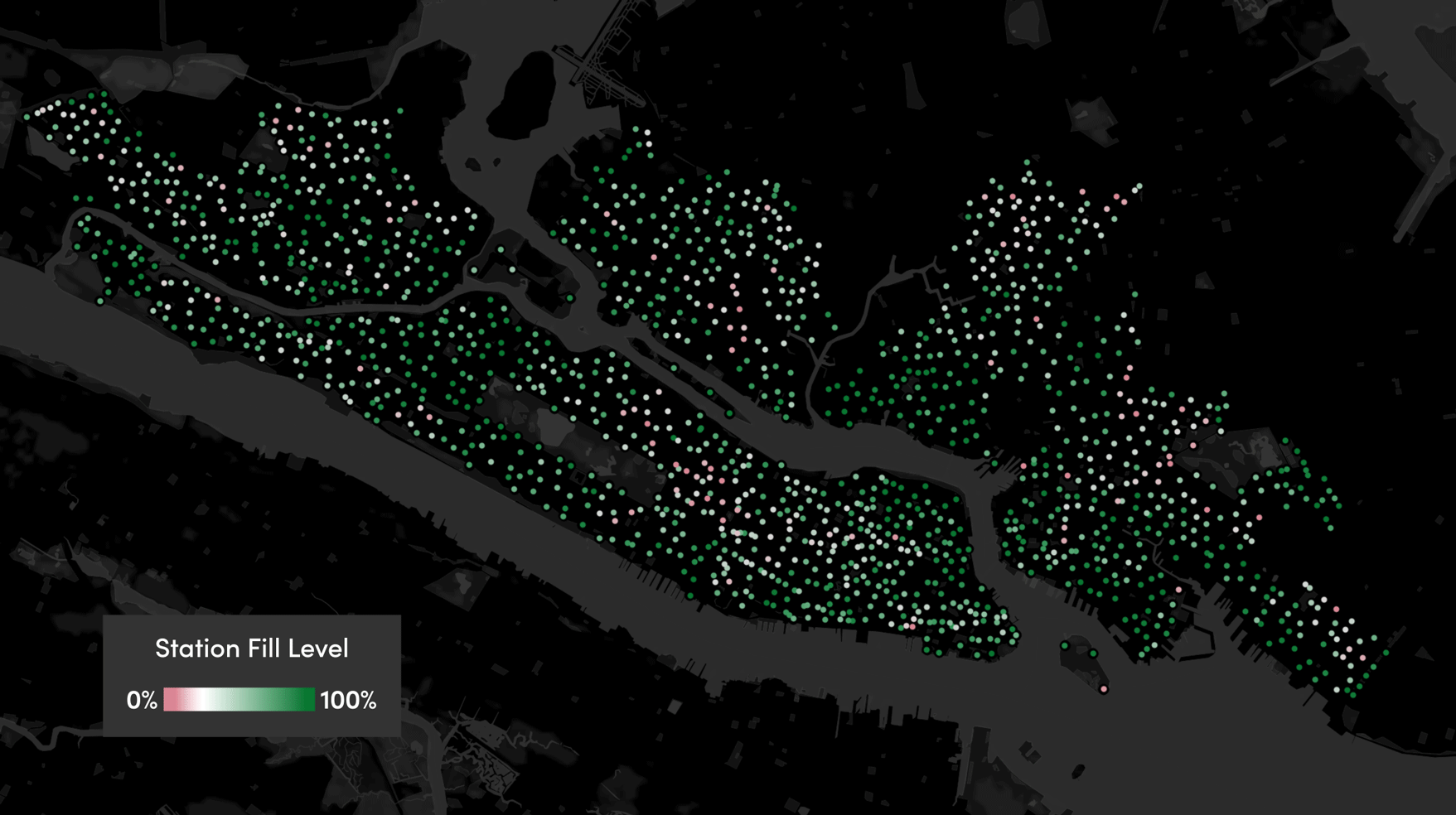
The output of all this number-crunching is relayed back to the operations team via a web application called AirControl, which maps, in varying shades of red and blue, the severity of actual (and predicted) demand or oversupply — and optimizes routes accordingly.
And just like a tide, there are distinct patterns. Bikes congregate below 34th Street in Manhattan. Neighborhoods underserved by other forms of transit, like Brooklyn’s Red Hook, also tend to collect bikes. Nightlife destinations like Williamsburg get a lot of inbound bikes from Manhattan. And bikes, like water, tend to flow downhill. “We’ll see parts of the Upper West Side where people love to ride a bike downhill to work, but no one’s bringing it back up,” Knoth says. On Sunday evenings, Citi Bike rebalancers are particularly busy, cleaning up the system for the workweek after a weekend of play.
Vans, valets, and Angels
From time to time, outside forces can upend bike-share behavior — like, say, a multiyear pandemic. Once-bustling areas, like Midtown and the Financial District, saw drops in ridership. And an increase in car traffic made rebalancing more challenging: Vans now spend up to 40% more time making trips, which are often, by necessity, made to the far edges of the system. The longer it takes bikes to arrive — or docks to be freed — the more potential trips are impacted. Lyft has responded by adding labor hours, more stations and docks, as well as valets, who accept bikes from riders when docks are fully occupied and release bikes from a nearby stockpile when demand is high, doubling or tripling the capacity of the busiest stations.
E-bikes add another twist. “E-bikes are a unique breed,” says Knoth. “We tend not to rebalance them because they move across the system so quickly.” They are in such high demand, Knoth says, that it doesn’t make sense to tie one up for an hour’s ride across town in a rebalancing van when it might make two or three trips during that period.
New York has one potent additional weapon in its rebalancing quiver: its Bike Angels program, which gives riders points (to be redeemed for free rides, among other things) for riding bikes to stations in need. “Bike Angels is more micro,” says Knoth, “like within a neighborhood.” The larger project of rebalancing happens on a borough-to-borough scale, and not many Angels would be willing to deliver a bike from deepest Brooklyn to northern Manhattan. “I’d have to offer a lot of points to get someone to do that ride,” Knoth says.
At the end of the day, one metric matters. “Everything,” says Stasko, “is examined through the lens of how many rides we think this will enable.” After all, a parked bike, like a parked car, is no longer transportation — it’s just street furniture.
Tom Vanderbilt is the author of Traffic: Why We Drive the Way We Do (and What It Says About Us) and Beginners: The Joy and Transformative Power of Lifelong Learning. His writing has appeared in Outside, WIRED, Slate, and many other publications.
The content provided in this article is for informational purposes only. Unless otherwise stated, Lyft is not affiliated with any businesses or organizations mentioned in this article.